Christopher Iliffe Sprague
Postdoctoral researcher at SciLifeLab
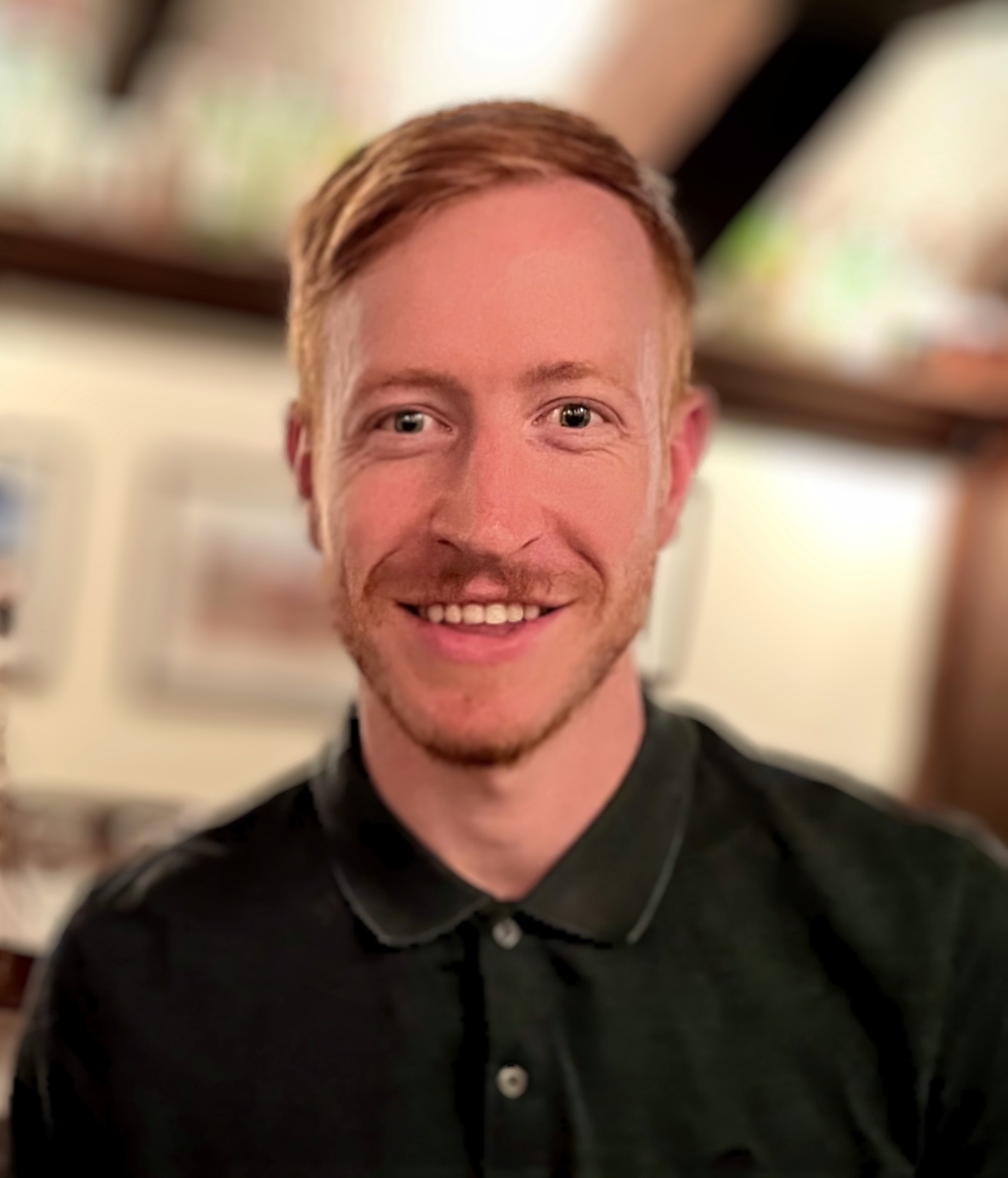
Robotics, Perception, and Learning
Teknikringen 14
Stockholm, Sweden, 114 23
Research
My research sits at the crossroads of learning-based and model-based methods. On one hand, I aim to optimise the efficacy of model-based methods using learning techniques. On the other hand, I work to ensure the reliability of learning-based methods through model-based approaches.
My core areas of interest include robotics, physical sciences, and life sciences. These fields offer a wealth of inductive biases that can enhance learning-based methods, especially in low-data environments.
Currently, I’m expanding my research horizons as a postdoctoral researcher at SciLifeLab, in collaboration with Prof. Hossein Azizpour and Prof. Arne Elofsson. I’m exploring the potential of learning-based methods in modelling molecular interactions. My areas of interest in this domain include protein-protein interactions, drug discovery, and protein folding. Despite the scarcity of data in this field, it boasts a rich supply of geometric and physical principles that can be used to enhance learning-based models.
Prior to my current role, I pursued my PhD at the KTH Royal Institute of Technology in Stockholm, Sweden, in collaboration with Prof. Petter Ögren. My doctoral research focused on crafting efficient and trustworthy AI solutions for critical robotic systems, using behaviour trees. This work underscored the importance of efficiency and safety while exploring the potential of learning-based methods to achieve these goals, all within a framework of model-based reliability.
Before embarking on my PhD journey, I obtained both my Master’s and Bachelor’s degrees at Rensselaer Polytechnic Institute in Troy, NY, USA, specialising in aerospace engineering.
News
Latest posts
Feb 9, 2024 | Stable Flow Matching |
---|